What is Federated Learning?
Federated learning is a way to train AI models without anyone seeing or touching your data, offering a way to unlock information to feed new AI applications.
The spam filters, chatbots, and recommendation tools that have made artificial intelligence a fixture of modern life got there on data — mountains of training examples scraped from the web, or contributed by consumers in exchange for free email, music, and other perks.
Many of these AI applications were trained on data gathered and crunched in one place. But today’s AI is shifting toward a decentralized approach. New AI models are being trained collaboratively on the edge, on data that never leave your mobile phone, laptop, or private server.
This new form of AI training is called federated learning, and it’s becoming the standard for meeting a raft of new regulations for handling and storing private data. By processing data at their source, federated learning also offers a way to tap the raw data streaming from sensors on satellites, bridges, machines, and a growing number of smart devices at home and on our bodies.
Federated Learning Based IDS.
For the development of systems and applications based on Machine Learning, this decentralized approach is very important, and not only that, but even this new approach also helps secure the privacy of end users.
So, it seems that the complete mechanism of this new approach, Federated Learning (FL), is comprehensive and advanced in nature, as the security systems can use this approach for better protection and defense mechanisms.
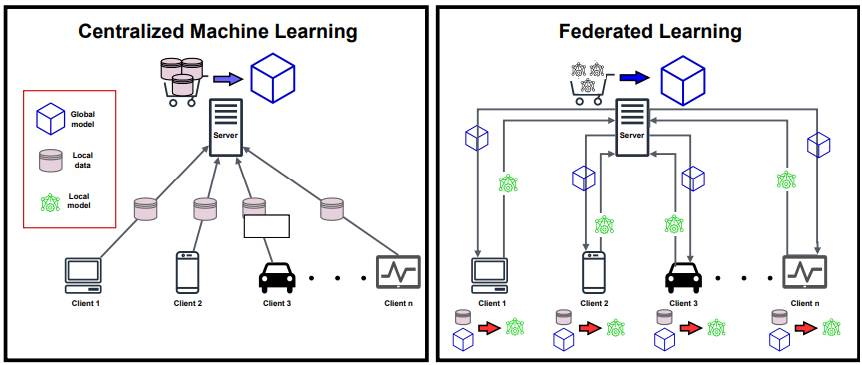
The IDS (Intrusion Detection System) detects the threats in IT/OT systems, and the FL-based IDS growth links to accurate evaluation datasets.
Besides this, the initial evolution of IDSs was mainly focused on the approaches that are signature-based, but the recent combination of IDS with an FL-based approach seems promising and far more efficient than the previous approaches.
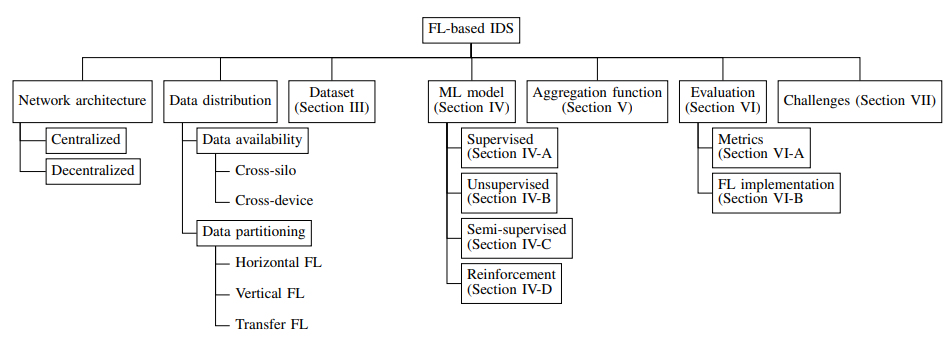
Here below, we have mentioned all the datasets:-
- CIC-IDS2017
- CSE-CIC-IDS2018
- CIDDS-001
- CIDDS-002
- CIC DoS
- PUF
- TRAbID
- Unified host and network
- N-BaIoT
- Kitsune
- Bot-IoT
- AWID3
- WUSTL-IIoT
- H23Q
- IoT network intrusion dataset
- IoTID20
- IoT-23
- IoT Healthcare Security Dataset
- e IoT DoS and DDoS Attack Dataset
- CCD-INID-V1
- X-IIoTID
- Edge-IIoTset
- MQTT-IoT-IDS2020
- MQTTset
- LATAM-DDoS-IoT dataset
- UAV Attack Dataset
- CIC IoT dataset
- Intrusion Detection in CAN bus
- Ton IoT dataset
- Car Hacking
- MedBIoT
- Car Hacking: Attack & Defense Challenge
- InSDN
- OTIDS
- Vehicular Reference Misbehavior Dataset (VeReMi)
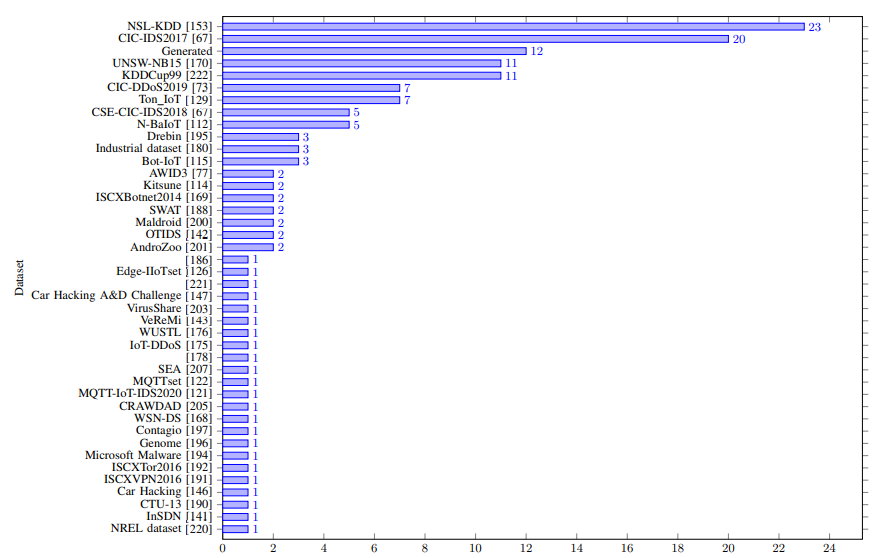
In this scenario, the neural networks (NNs) used in IDS match the two key elements, and here below, we have mentioned them:-
- Input features
- Classes
Moreover, the RL (Reinforcement Learning), an ML branch, has agents learn to maximize rewards through trial and error. While it categorizes into the following methods:-
- Value-based
- Policy-based
- Model-based