What is trusted AI?
Trusted AI means avoiding unwanted side-effects. These might include physical harm to users, as in the case of AI accidents, or more intangible problems like bias.
Microsoft outlines six key principles for responsible AI: accountability, inclusiveness, reliability and safety, fairness, transparency, and privacy and security. These principles are essential to creating responsible and trustworthy AI as it moves into mainstream products and services. They’re guided by two perspectives: ethical and explainable.
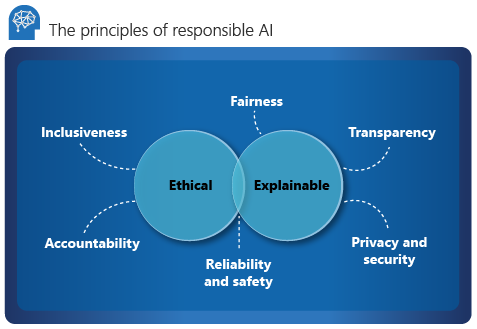
Now we know the each one of the two principles.
Ethical :
From an ethical perspective, AI should:
- Be fair and inclusive in its assertions.
- Be accountable for its decisions.
- Not discriminate or hinder different races, disabilities, or backgrounds.
1. Accountability
Principle: People must maintain responsibility for and meaningful control over AI systems.
Last but not least, all stakeholders involved in the development of AI systems are in charge of the ethical implications of their use and misuse. It is important to clearly identify the roles and responsibilities of those who will hold accountability for the organization’s compliance with the established AI principles.
There’s a correlation between the degree of complexity and autonomy of the AI system and the responsibility intensity. The more autonomous AI is, the higher the degree of accountability of the organization that develops, deploys, or uses that AI system. That’s because the outcomes may be critical in terms of people’s lives and safety.
2. Inclusiveness
Inclusiveness mandates that AI should consider all human races and experiences. Inclusive design practices can help developers understand and address potential barriers that could unintentionally exclude people. Where possible, organizations should use speech-to-text, text-to-speech, and visual recognition technology to empower people who have hearing, visual, and other impairments.
Reliability and Safety:
Principle: AI systems should operate reliably, safely, and consistently under normal circumstances and in unexpected conditions.
Organizations must develop AI systems that provide robust performance and are safe to use while minimizing the negative impact. What will happen if something goes wrong in an AI system? How will the algorithm behave in an unforeseen scenario? AI can be called reliable if it deals with all the “what ifs” and adequately responds to the new situation without doing harm to users.
To ensure the reliability and safety of AI, you may want to
- Consider scenarios that are more likely to happen and ways a system may respond to them,
- Figure out how a person can make timely adjustments to the system if anything goes wrong, and
- Put human safety first.
Explanable :
Explainability helps data scientists, auditors, and business decision makers ensure that AI systems can justify their decisions and how they reach their conclusions. Explainability also helps ensure compliance with company policies, industry standards, and government regulations.
A data scientist should be able to explain to a stakeholder how they achieved certain levels of accuracy and what influenced the outcome. Likewise, to comply with the company’s policies, an auditor needs a tool that validates the model. A business decision maker needs to gain trust by providing a transparent model.
1. Fairness
Principle: AI systems should treat everyone fairly and avoid affecting similarly-situated groups of people in different ways. Simply put, they should be unbiased.
- Research biases and their causes in data (e.g., an unequal representation of classes in training data like when the recruitment tool is shown more resumes from men, making women a minority class);
- Identify and document what impact the technology may have and how it may behave;
- Define your model fairness for different use cases (e.g., for a certain number of age groups); and
- Update training and testing data based on the feedback from those who use the model and how they do it.
2. Transparency, interpretability, and explainability
Principle: People should be able to understand how AI systems make decisions, especially when those decisions impact people’s lives.
AI is often a black box rather than a transparent system, meaning it’s not that easy to explain how things work from the inside. After all, while people sometimes can’t provide satisfactory explanations of their own decisions, understanding complex AI systems such as neural networks can be difficult even for machine learning experts.
While you may have your own vision of how to make AI transparent and explainable, here are a few practices to mull over.
- 1. Decide on the interpretability criteria and add them to a checklist (e.g., which explanations are required and how they will be presented).
- 2. Make clear what kind of data AI systems use, for what purpose, and what factors affect the final result.
- 3. Document the system’s behavior at different stages of development and testing.
- 4. Communicate explanations on how a model works to end-users.
- 5. Convey how to correct the system’s mistakes.
3. Privacy and Security
A data holder is obligated to protect the data in an AI system. Privacy and security are an integral part of this system.